AI was born during a small, Dartmouth College workshop, in 1956, where a handful of scientists demonstrated a simple “thinking” program that would eventually prove 38, of the first 52, theorems in Whitehead and Russell’s Principia Mathematica.
Since then, the AI field has experienced ups and downs, until 2009, when silicon valley executives started using Nvidia GPUs (instead of CPUs) to increase the speed of computation by about 100 times and ignited the Big Bang of deep learning and modern AI.
Now multiple organizations are tracking the developments in AI by measuring investments, technical progress, research citations and university enrolments.
A recent PwC research report, an Accenture paper, a McKinsey Global Institute publication and a National Bureau of Economic Research article all confirmed AI as the biggest commercial opportunity in today’s economy.
PwC reported that by 2030 the accelerating development of artificial intelligence will push world the GDP 14% higher or an additional $15.7 trillion (equivalent to total European 2016 GDP).
The greatest gains from AI are likely to be in China (26% boost to GDP in 2030) and North America (potential 14% boost).
The biggest sector gains will be in retail, financial services and healthcare as AI increases productivity, product quality and consumption. According to recent numbers the economic impact of AI will be driven by:
Automating processes (including use of robotics and autonomous vehicles).
Productivity gains from businesses augmenting their existing labour force with AI technologies (assisted and augmented intelligence).
Increased consumer demand resulting from the availability of personalised and/or higher-quality AI-enhanced products and services.
New applications every day
AI seems to be disrupting a new industry every week and is taking an increased role in our day-to-day lives. In fact by early 2019 AI is projected to be in almost all electronic devices we use.
Even though AI functionalities are currently expensive and complex, over the next few years AI will become increasingly more accessible and will lead to the automation of many of things currently done manually.
AI is already used to track world’s wildlife, it predicts IQ from brain scans, it can understand volcanic eruptions and solve the email overload.
AI is also disrupting the ETF industry and is having a spillover effects into the rest of the financial sector.
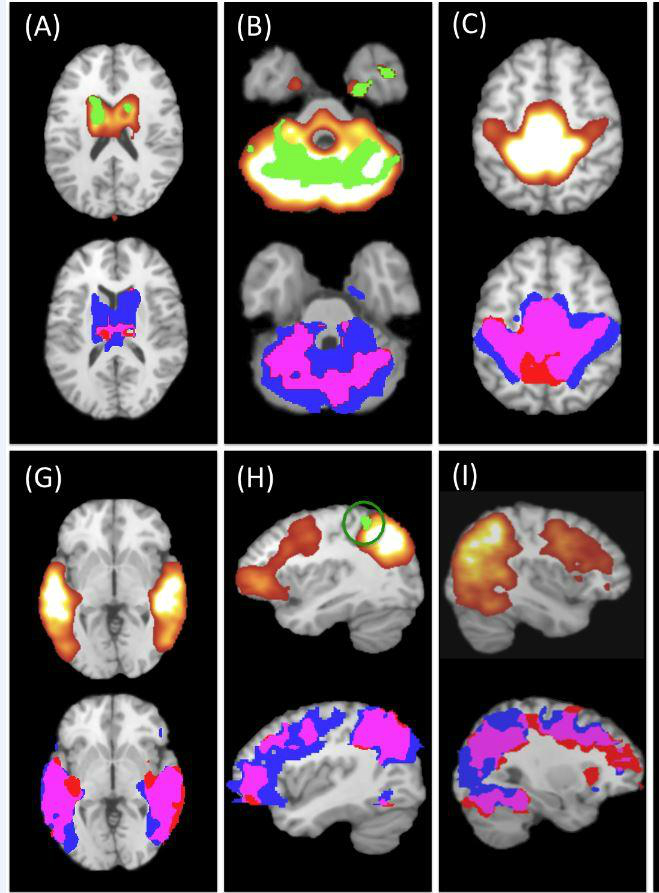
Many, usually not very vocal hedge fund managers are admitting to using AI to track millions of news articles, social media posts and market signals.
From Universities to Nvidia GPUs
Since its unofficial birthday at a Dartmouth workshop, in summer 1956, artificial intelligence has experienced waves of optimism followed by periodic phases of disappointment and loss of funding known as AI winters (1974–1980 & 1987–1993).
In fact until the early 2000s, most researchers deliberately called their work by other names, such as informatics, cognitive systems or computational intelligence for fear of being viewed as sci-fi dreamers.
However, even though AI received little to no credit for multiple accomplishments in the 1990s, AI based algorithms began to solve lot of very difficult problems such as Google’s search engine, data mining, industrial robotics, speech recognition and medical diagnosis.
By 2003, deep learning (DL) became part of state-of-the-art systems in various disciplines, particularly computer vision and automatic speech recognition and was seriously impacting the industry.
According to Yann LeCun, convolutional neural networks (CNNs) were already processing an estimated of 10% to 20% of all the cheques written in the US.
The Big Bang happened in 2009 when Andrew Ng, founder of Google Brain, figured out that Graphics Processing Units (GPUs) increase the speed of deep-learning systems by 100 times and used Nvidia video cards to create capable deep neural networks (DNN).
Turned out that GPUs, originally designed for graphic-intensive games, are perfectly-suited for the matrix/vector math involved in machine learning and can speed up training algorithms by orders of magnitude, reducing running times from weeks to days.

How machine learning algorithms learn
Most machine learning algorithms, Neural Networks (ANN), Convolutional Neural Networks (CNN) and Recurrent Neural Networks (RNN) are computing systems vaguely inspired by the biological neural networks that constitute animal brains.
They learn to perform tasks, generally without being programmed with any task-specific rules by automatically generating identifiable characteristics from the learning material that they process.
All neural networks and deep learning algorithms perform complex statistical computations, from predicting financial data to identifying a picture of a dog everything is first decoded into a set of multi-dimensional matrix constituted by numbers.
If we take an image of a dog for example, the first step is to turn it into the grayscale format and then to assign a matrix to each pixel depending on how light or dark it is.
A simple mobile phone picture is translated to few million pixels, which is in turn converted to a large matrix of numbers. These matrices of numbers are fed as input into the neural network along with a detailed classification label.
Training a neural network with thousands of dog images, is going to produce a model that can easily recognize a visible dog in a picture. This training process is all about correlating multiple pixels (numbers) with “dog” label so it can identify patterns of a dog from a test image.
The classification process involves multiplying millions of matrices with each other to arrive at the proper correlation. Typical CPUs are designed to perform calculations in a sequential order, meaning mathematical operation will have to wait for the previous calculation to complete and CPUs with multiple cores are very expensive, making them less optimal for training neural networks.
On the other hand, GPUs designed for graphic rendering, have a processor with thousands of cores capable of performing millions of mathematical operations in parallel and can process a huge number of matrix multiplication per second.
That’s one of the reasons why scientists prefer high-end GPUs for deep learning. Nvidia has came up with a programming model for GPU called CUDA (Compute Unified Device Architecture) and another class of GPU called GPGPU (General-Purpose GPU) designed for parallelizing computation.
With CUDA, AI researchers and developers can send Forta, C and C++code directly to the GPU without using assembly code.
Now to put things in perspective, Nvidia’s latest GTX 1180 GPU will pack 3,584 CUDA cores while Intel’s top end server CPUs may have a maximum of 28 cores.
Artificial Intelligence and Machine Learning are changing the landscape of enterprise IT. The recent interest in GPUs is attributed to the rise in AI and cryptocurrency mining.
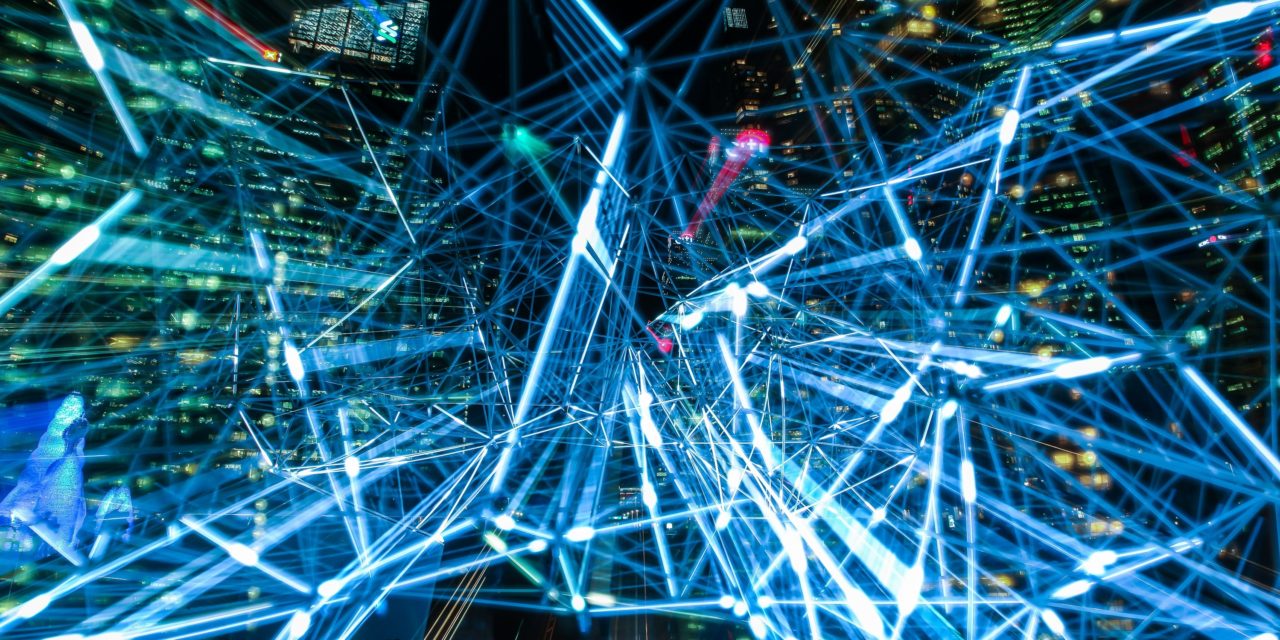
Enters Blockchain
Since we have moved from an industrial to an information-based economy, formed around global and digital interactions, we entered a time of unregulated and ungoverned data usage.
Large-scale fraud, identity theft and data breaches are becoming common as commoditization and monetization of personal information is increasing and massive datasets are progressively getting locked up into few centralized silos.
Human inherent desire for privacy and the vulnerability of the existing identity management methods are key drivers of the current shift towards decentralization.
Blockchain, a new foundational technology, holds the potential to reduce the risk of unauthorized access and data manipulation through strong encryption and tamperproof audit trails.
At this moment blockchain holds the potential to break down current barriers of AI development that could lead to more efficiency and greater openness, with lower costs.
As of July 2018, several jurisdictions in the U.S. have amended their state laws to explicitly legitimize the use of blockchain technology in both commerce and corporate governance, and introduce a historic immutable record of activity leading to greater data veracity,
Delaware amended the Delaware General Corporation Law (DGCL) to expressly allow Delaware corporations to use blockchains to create and maintain corporate records.
Blockchain based AI platforms and applications are currently setting up a framework for trusted data. As of today NBAI is a leader in blockchain based AI computing resource distribution and has the ability to cut computing costs by 70% when compared to traditional centralized cloud computing providers.